How to AI?
How to AI? or How can I use AI? is a question that resonates with many business leaders as the world continues to embrace technology. The utilization of AI has enabled us to process data more efficiently and make informed decisions with greater accuracy. So, I wanted to delve into how you can leverage AI tools in your business endeavors.
We’ll explore how Large-language-models are changing the landscape of predictive analysis and decision-making, respectively. You’ll understand why quality data acquisition is paramount for effectively utilizing these technologies.
Furthermore, we’ll shed light on cognitive technologies such as natural language processing (NLP) and their role in enhancing business operations. We will also discuss practical ways through which businesses have successfully integrated these technologies into their existing systems.
Lastly, How can I use AI? It wouldn’t be completely answered without discussing the application AI. Learn about innovative platforms that utilize AI algorithms while ensuring personalized engagement with customers.

Table of Contents:
- How to AI?
AI Strategy
The path to becoming an organization powered by AI begins with developing a well-defined AI strategy that aligns with your business objectives. Before diving into the hype surrounding artificial intelligence, it is crucial to understand the complexities of this technology. Nevertheless, you don’t have to wait until you have everything fully developed; simply start implementing and exploring the possibilities.
Identify objectives from your corporate strategy that are digitally driven, assess if there are AI use cases that can help you achieve those objectives, and then start testing it out in small steps.
Understanding the Data
The first step in leveraging AI is understanding the journey of data from acquisition to usage. Collect, analyze, interpret, repeat. It’s like having a magical window into the future, using past information as an oracle.
Importance of Quality Data Acquisition
Garbage in, garbage out. To make AI work its magic, we need top-notch data. No room for errors or missing pieces. So, invest in tools and technologies that keep your data clean and accurate. And remember, consistency is key. From start to finish, maintain that data integrity.
Luckily, a few solutions, such as OpenAI GPT, BERT from Google, and many others, are already pre-trained on generally available data. That means that businesses will be able to benefit from their AI models, however, not in a specialized form, e.g., AI for Healthcare, and not in an individualized manner, e.g., AI based on your company’s data.
The Journey of Data
The journey begins with the acquisition, where raw data is collected and stored. This can be anything from user behavior on a website to sensor readings in an industrial setting. The next step involves cleaning this raw data – removing outliers or errors and filling in any missing values. Once cleaned, the processed datasets form the basis for training the AI solution both technically and functionally.
- Data acquisition: Gather data from internal resources such as contracts, operation manuals, and business process descriptions, and external resources such as websites, social media, and more.
- Data analysis: Crunch numbers to find the juicy bits.
- Predictive modeling: Use fancy math to find patterns, predict the future, or generate new data.
AI algorithms learn from data
It’s crucial to understand that, at its core, AI is about data. In fact, one could argue that AI is essentially an advanced form of data analysis. AI uses datasets to train models by finding patterns within them using different types of algorithms like classification or regression. For instance, chatbots can’t answer queries without proper data.
Understanding Artificial Intelligence
The hype surrounding artificial intelligence has led several organizations astray into pursuing unsuitable technologies for their needs—resulting in wasted time and resources. With a comprehensive understanding of different technologies such as machine learning or natural language processing, you are better positioned to identify your specific requirements, select appropriate vendors, and estimate implementation timelines accurately.
Machine learning – a subset of AI – uses algorithms – essentially sets of rules or instructions – that allow computers to learn from data without being explicitly programmed. Deep learning takes this concept further by using neural networks modeled after the human brain for more complex tasks like visual recognition systems.
It is crucial to understand the strengths and limitations of various technologies before embarking on an AI initiative. Rule-based expert systems and robotic process automation (RPA), for instance, provide transparency in their operations but lack learning capabilities. In contrast, deep learning and LLMs (Large-language-models) excel at processing large volumes of labeled data; however, their “black box” nature can be problematic.
Businesses today are overwhelmed by the amount of data available. But fear not. With AI algorithms, we can dive deep and find hidden treasures. These insights help businesses make smarter decisions by predicting what’s coming next. Cognitive technologies like Natural Language Processing (NLP) and deep learning are changing the game in business. These AI components allow machines to understand human language and mimic human intelligence.
The Role of NLP in Business Operations
Natural Language Processing (NLP) combines computing, AI, and linguistics to enable computers to comprehend human language. No more specific commands – now businesses can automate customer service with chatbots and analyze social media sentiment for marketing insights. NLP enables effective customer interactions and data-driven decision-making. An example here would be NLP Cognitive-chatbots. Those intelligent agents learn from past interactions to improve future responses, thereby providing personal advisor services. Additionally, deep learning visual recognition systems are also gaining popularity due to their ability to identify objects in images and videos with high accuracy and precision, making them invaluable tools in many industries, including healthcare, security, agriculture, and more. So if you’re looking to recruit expert in-house talent to enhance your organization’s capabilities, consider investing in these areas as they promise a significant return on investment in the long run.
Deep Learning’s Impact on Decision Making
Deep learning, inspired by the human brain, uses neural networks to learn from vast amounts of data at lightning speed. Deep learning has revolutionized decision-making within organizations. The real power lies in its ability to recognize patterns in large datasets that humans can’t. Deep learning predicts future sales trends and identifies potential risks, giving businesses foresight into outcomes.
To truly appreciate these cognitive technologies, we must understand their practical applications in various industries. From healthcare to finance and retail, NLP and deep learning revolutionize disease detection, fraud detection, and personalized recommendations.
Adopting cognitive technologies isn’t just about staying ahead technologically – it’s about surviving fierce competition by leveraging AI’s transformative powers efficiently.
Cognitive AI Applications
In addition, cognitive AI applications are another proposed AI solution that is both technically and strategically significant for businesses today. They provide actionable insights based on patterns identified through data analysis, which, when integrated into existing systems, could automate processes previously handled by humans – much like earlier business-process automation tools did.
Finding Suitable Tools
Your choice of software will be largely determined by what you aim to achieve with artificial intelligence within your business operations. For instance, if you’re looking at automating repetitive tasks previously done manually (akin to earlier business-process automation tools), then RPA (Robotic Process Automation) platforms would be suitable choices.
If it’s about leveraging cognitive insight applications, then solutions featuring intelligent agents may serve a better purpose. Software frameworks designed specifically for machine learning projects, such as TensorFlow or PyTorch, could prove useful, too, especially when dealing with more sophisticated use cases involving conducting extended unstructured dialogues or automated advice services.
Uncovering the Right Hosting for your AI
Choosing the appropriate hosting solution for running AI models is crucial in embarking on the path to becoming an organization powered by artificial intelligence. This entails identifying the necessary hardware and software components capable of executing intricate AI algorithms, machine learning models, and data analysis tasks. It’s important to note that not all existing systems can handle the processing power required by deep learning visual recognition systems or natural language processing chatbots. These advanced technologies demand high-performance processors, large memory capacities, and fast storage solutions.
In addition to this, you might also want to consider investing in specialized equipment like GPUs (Graphics Processing Units), which are known for their ability to process multiple computations simultaneously – a feature highly beneficial when training large neural networks. Another option is to leverage AI-as-a-Service platforms available in the cloud. These platforms offer AI capabilities without requiring internal expertise or infrastructure.
AI Talents
Remember, though, while there is a lot of hype surrounding artificial intelligence today, which has led to an increased demand for experts in this field, it’s crucial you ensure having the right experts with the right skills.
A typical AI-powered organization would require data scientists, AI specialists, and IT professionals. These individuals must be adept at using various AI tools and algorithms to create solutions that meet organizational needs. For instance, they could develop a proposed AI solution both technically and strategically within your organization’s existing systems.
- Data Scientists: They perform data analysis as well as design and implement models based on those analyses. As technology improves over time, their role becomes increasingly important because they help organizations make sense out of large amounts of data.
- AI Specialists: Their main job is developing intelligent applications using advanced techniques such as NLP, deep learning and visual recognition systems.
- IT Professionals: IT professionals ensure smooth integration between different parts of an organization, thereby enabling seamless communication among them.
In-house talent who understand these technologies well enough can guide you through your digital transformation journey effectively without getting lost amidst all the technological jargon flying around!
However, forcing a former Database administrator, an in-house Python developer, or a PowerBI expert to be a Machine Learning Engineer overnight, can only cost you a lot of time and money later on your journey. So take time to select, train, and augment your team with the right resources without relying solely on external consultants or vendors.
These talents need expertise in areas like machine learning, deep learning, and natural language processing, which are all part of the broader field of computer science. They should also have experience with earlier business-process automation tools since these form the foundation upon which modern-day AI programs are built.
Leveraging Key Employee Capabilities
Your team’s willingness to learn new concepts plays a significant part here. While some might leap at opportunities offered by advancements like cognitive insight applications or deep learning visual recognition systems, others may prefer sticking with familiar tools. It is essential that you encourage curiosity among your staff members so they can adapt quickly when needed.
Implementing AI
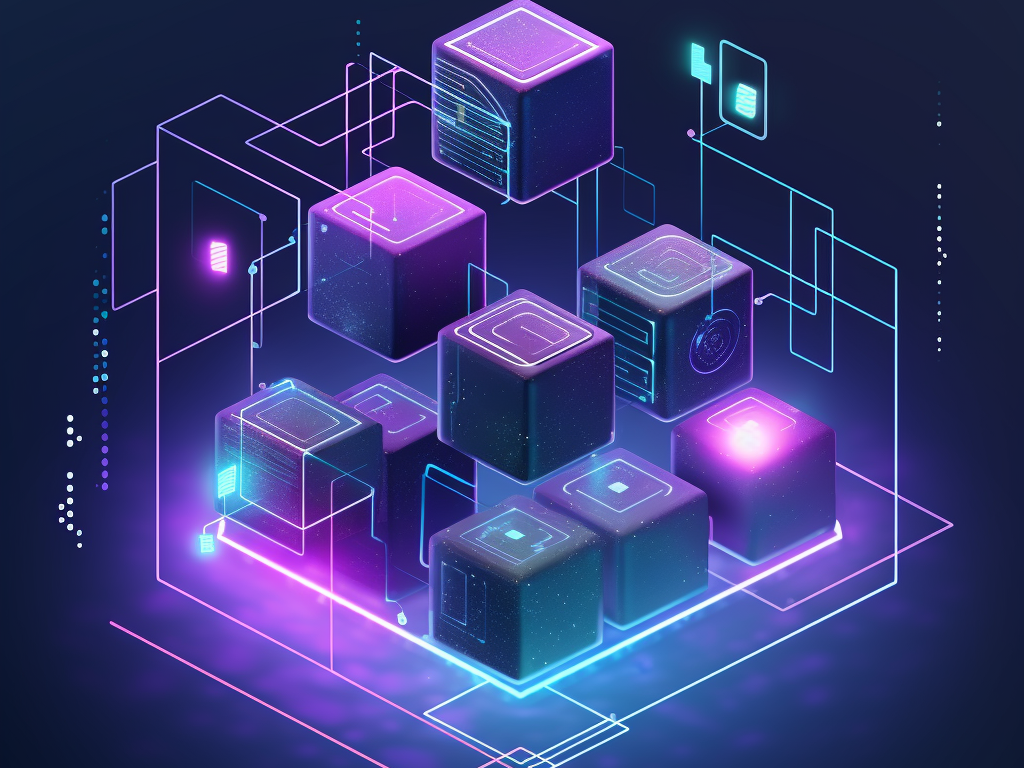
Piloting with Purpose
In the world of artificial intelligence (AI), launching pilots is an essential step to bridge the gap between current and desired AI capabilities. This approach allows companies to create proof-of-concept projects for cognitive applications before a full-scale rollout.
Proof-of-concept pilots are particularly effective when applied to initiatives that offer high-potential business value or allow organizations to test various technologies simultaneously. However, it’s crucial not to bypass this rigorous piloting process under pressure from senior executives who technology vendors may influence. Injected projects often fail, setting back the organization’s AI program.
Cognitive Centers of Excellence
If your company plans on launching several pilots, consider creating a cognitive center of excellence or similar structure for management purposes. This strategy helps build necessary technology skills within the organization while transitioning small pilot programs into broader applications for greater impact.
Pfizer, for example, employs some form of cognitive technology in more than 60 projects across their enterprise; many are still in pilot stages, while others have already entered production.
Becton Dickinson, another leading firm in this space, has adopted a “global automation” function within its IT department, overseeing numerous cognitive tech pilots employing intelligent digital agents and RPA (Robotic Process Automation). They use end-to-end process maps as guides during implementation and identifying automation opportunities along with graphical “heat maps” indicating organizational activities most amenable to AI interventions.
The health insurer Anthem has developed a centralized AI function called Cognitive Capability Office following similar lines which reflects how these firms are leveraging AI tools like natural language processing chatbots and deep learning visual recognition systems successfully launched through their respective cognitive pilots.
Scaling Up
The journey from a successful pilot to full-scale implementation can be daunting. Many organizations have launched cognitive pilots with promising results, but scaling up these initiatives across the entire organization has proven more challenging. Implementing AI at scale is like teaching a robot to do the Macarena – it’s no small feat. It requires a big investment of time, money, and brainpower. But fear not; some companies have cracked the code and successfully integrated AI into their operations.
The key to success lies in detailed planning and collaboration between technology experts and business process owners. Since these technologies often support individual tasks rather than whole processes, integration with existing systems is crucial for scale-up. This way, companies are better positioned to overcome challenges associated with integrating artificial intelligence into their existing infrastructure—identified as one of the major hurdles —and make strides towards becoming an efficient, future-ready AI-powered organization.
Overcoming Financial and Technical Challenges
Implementing AI on a large scale can be a real money guzzler. You must invest in data storage, processing power, and talent acquisition. Plus, you need brainiacs who can develop and maintain those complex algorithms.
To tackle these challenges, businesses must invest in a rock-solid infrastructure that can handle big data, and they should encourage their employees to keep learning about the latest machine learning advancements. It’s like giving them a ticket to the AI rollercoaster.
Conclusion
How can I use AI? It has become an essential question for many businesses since AI is an integral part of our daily lives. However, many are still unsure how they can leverage this technology effectively. But always remember, the goal is not just to adopt AI for the sake of It but to use it as a tool to enhance business outcomes.
Understanding the journey of data and exploring cognitive technologies are key steps in utilizing AI effectively.
Leveraging machine learning for predictive analysis and acquiring quality data is essential for making informed decisions.
Natural language processing plays a crucial role in improving business operations, while deep learning enhances decision-making processes.
Leveraging AI-as-a-Service, many cloud-based platforms provide AI capabilities without requiring you to recruit expert in-house talent or infrastructure. These platforms offer tools like machine learning, deep learning visual recognition systems, and natural language processing that can enhance your business operations.
Outsourcing is an effective solution if building an internal team seems daunting. External partners with expertise in AI algorithm development can help implement your proposed AI solution both technically and strategically—ensuring quick and effective results.
A Small Start Can Lead To Big Wins. No need to jump into large projects immediately! Starting small allows you time for data analysis while reaping the benefits of earlier business-process automation tools.
Increase your team’s skill set. Your existing team members are valuable assets – invest in them! There are countless online courses available that cover everything from basic computer science concepts to advanced topics such as deep learning and natural language processing. Coursera, for instance, offers comprehensive training programs tailored toward various skill levels.
Focus on process improvement rather than chasing every hype surrounding artificial intelligence. Focus on improving what already exists within your organization first. Streamlining current processes could potentially yield significant gains before any new technology improves upon them further.